Resistance and Avalon Strategy: Analysis of an AI Tournament and Academic Research
How to win friends and influence people to not fail missions
(Previous AI strategy simulations of board games: Mexican Train, Uno/Crazy Eights, Love Letter.)
I think Avalon is the best hidden identity game ever created, so much so that I ran an AI tournament for it, and wrote up some thoughts on the state of AI research around it given a recent academic paper.
Background
You can find Avalon’s rules here, I would advise watching at least one game on video to get a sense for how it plays out if you haven’t played a comparable hidden identity game before, like Mafia or Werewolf. The core mechanics are:
Each player gets a hidden role which defines their team: Resistance wants missions to succeed, Spies want missions to fail, and Resistance has the majority.
Some roles get secret information: Spies learn who each other are, and a resistance role named Merlin learns who the Spies are.
The group decides by democratic consensus which players should attempt a mission, and those players can secretly submit success or fail cards.
The group learns how many fails were played, and repeats step 3-4 until three missions have succeeded or failed.
If three missions have failed, Spies win, if three missions succeed, the Spies get one final chance to guess Merlin to win; if they guess wrong, they lose.
Avalon has a few great properties that blow the competition away:
Unlike Mafia/Werewolf, there’s no player elimination; you’re in until the end of the game
With the Merlin/Assassin variant in Avalon, both teams have something to hide and something to figure out, even if your identity is known you can still be trying to solve the puzzle up until the last minute. Merlin knows the Spies and is trying to help the other Resistance players identify them, but if the Assassin can guess which player is Merlin at the end, the Spies still win.
You can add incremental complexity with extra roles to make the game more interesting, see the knowledge graph below
It leads to great mind-games like the following recent game I played:
Mark is the Assassin, trying to guess the identity of the character Merlin at the end of the game, who knew all the Spies. Mark mentions to the other Spies that Henry went out of his way to avoid having him on a team, and a battle of wits begins. Henry blurts out: "Oh, actually the reason I did that was because... oh shoot, I shouldn't talk to help the Spies." This is, of course, a classic Merlin quadruple-bluff gambit.
Bluff Level One: Henry could have genuinely forgotten he shouldn't talk in the endgame when it can only help the Spies guess who Merlin is, and accidentally made an admission that only a non-Merlin player would make, so Mark should conclude Henry is not Merlin.
Bluff Level Two: Henry correctly deduced that Mark would not believe for a minute that Henry would make a mistake like Level One, therefore Henry must have made the statement on purpose. Because the naïve interpretation of the statement makes it less likely Henry is Merlin, he must actually be Merlin trying to pretend to have different reasons than his actual reasons, so Mark should guess Henry as Merlin.
Bluff Level Three: Henry correctly deduces that Mark would not believe that Henry would make a mistake like Level One, and also deduces that Mark would correctly deduce that Henry would expect him to see the action as deliberate. This means both parties are aware that their counterpart knows that Henry planted the statement as a trap, meaning we can not be in Level Two, where one party hoped the other was unaware of the trap being set. Once you know a trap was planted with the expectation of being spotted, the obvious conclusion is that Henry leaked the statement on purpose to look like a Merlin trying to avoid suspicion, so Mark should not guess Henry as Merlin.
Bluff Level Sixteen: Mark starts with the initial conditions of the universe and quickly constructs a high fidelity simulation leading up until the present moment and deduces, perhaps incorrectly, that Henry was self-evidently planting a fifteen level-deep bluff to convince Mark that he was not Merlin, and that the winning play is to go sixteen levels deep and see through the bluff, so Mark correctly assassinates Henry for the win.
The complexity of the hidden information characters are trying to uncover and share can also be ratcheted up, as seen in the following diagram (this diagram skips the Morgana Spy role, which gives Percival another ambiguous option for who Merlin could be):
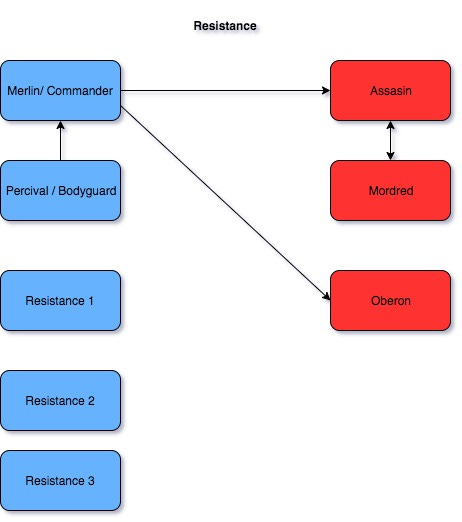
The game can also be played with the base Resistance set with no special roles other than the Spies, with Plot cards that give information to team leaders at different points of the game, and with some extra modules like the Hunter module, but the Avalon variant is the most balanced and strategically deep of the variants, in my experience.
Real-life strategy
Most of this piece is going to be spent talking about what we can learn about the game from AI simulations exploring its fundamentals, which is more objective, but also limited in what we can say definitively.
As I’ve played the game for 8+ years now myself, these are my high-level thoughts on real-life strategy.
Assume the game is won by Spies by default, and play accordingly.
Based on the simulations conducted and my own experience, I believe that the Resistance team is at a significant disadvantage without taking advantage of the social aspects of the game. The odds are against them forming a team without Spies by random chance, or having enough data from mission failures to lock onto a perfect team.
The Resistance team’s edge comes from effectively exploiting information, by Merlin nudging them towards accurate consensus without being caught, or by catching social tells from the Spies.
As Resistance, you should expect that a lot of conversation and speculation will be required to help your team win the game: the more free-flowing discussion there is, the easier it is for Merlin to drop hints, the more likely the team is to form an accurate consensus, and the more likely a Spy is to mess up under pressure. As a Spy, you can win the game a decent share of the time just by not getting caught, as the game can go off the rails just from Spies reliably failing missions. And when a game is going well, you will want to trace back why.
All that is necessary for the triumph of evil is for good Resistance players to do nothing.
Get used to mentally tracking the details of proposals, votes, and team outcomes. Also discussion, but hard data frequently beats social tells.
Based on simulations and my own experience, tracking simple metrics like which Resistance member had the most accurate votes is extremely useful for Spies trying to find Merlin, and keeping tabs on who has been most accurate or nudging the game in the wrong direction can help the Resistance team exclude Spies or form consensus around Merlin.
Social tells are also valuable, but some players are excellent actors, and hard data on what happened in the game should often be trusted over your own intuition: if a player is voting close to perfectly while being extremely convincing at acting confused, it’s often worth taking the data over your gut and picking them as Merlin.
As a Spy, often the most dangerous thing you can do is let a player know with 100% certainty you’re a Spy.
It both simplifies the puzzle for Resistance and risks empowering a player against you if your actions result in that player knowing for a fact you’re a Spy. This can happen when a Spy fails a two person mission with a Resistance player, a Spy fails alongside another Spy to give Resistance excellent information about the number of Spies on a team, or using variants like Lady of the Lake where Spies can falsely claim they saw a Resistance member was a Spy using the token’s ability.
It’s generally safer for Spies to fail larger missions and act in ways that maximizes ambiguity: having to win a confrontation against a player who has complete conviction you’re a Spy can be harder than being one of three suspects and playing them off against each other.
As Resistance, the most valuable thing you can do is solve the puzzle, and provide cover for Merlin.
Based on simulations and my own experience, Merlin operates best when they have a team of Resistance players acting like Merlin would themselves: voting accurately, calling out Spies for being suspicious, defending players who are innocent, and working to build consensus. If Resistance simply waits around for Merlin to save them, the Spies’ decision in guessing Merlin will be obvious. The best thing you do is help influence the game in the right direction and give Merlin some room to back up your theories, so Merlin doesn’t always have to be the first one to speak.
Think about and practice how to play each role.
Each role comes with a different set of information and a different puzzle to solve: Merlin wants to influence the game without being obvious, Percival wants to draw the Spies’ attention away from Merlin, base Resistance wants to solve the puzzle quickly and look like they have inside information, Oberon wants to avoid failing alongside other Spies and support unknown Spy allies, Assassin wants to track which Resistance player is acting like Merlin, Morgana wants to fool Percival and trace back who Merlin is, and Mordred wants to exploit Merlin’s ignorance of their role to remain hidden until the end.
The better you understand each role, the better you’ll be able to guess when someone else is playing it, and the better you’ll be at adapting your playstyle to cover each option: you can sometimes be an effective Spy just by being quiet, but that will likely hold your team back as Resistance.
There’s also a 45-page guide on BoardGameGeek which dives into the social aspect of the game. My own opinions differ on some points, and I also think strategies depend heavily on your group context/meta.
Harvard/MIT’s DeepRole Avalon AI, and its suboptimal plays
An excellent academic paper on Avalon strategy was published in 2019 titled Finding Friend and Foe in Multi-Agent Games, analyzing 5 player Avalon with Merlin and Assassin as the special roles, written by a combination of students and professors at Harvard and MIT. Their paper has a number of interesting results, and shows that AIs can co-ordinate to achieve accurate consensus in complex gameplay situations. The paper describes its best AI as being superior to human players, but I’m confident their AI makes some mistakes human players could avoid, for reasons I’ll discuss shortly.
Highlights from their paper:
Their AIs ignore the impact of “Cheap Talk”, namely any form of communication that’s not in the form of actions that can impact the game: their AI reaches conclusions based only on team proposals, votes, and mission results. I believe that optimal play would take advantage of “Cheap Talk”, for reasons I’ll discuss later.
Even at 5 players, Avalon has an enormous state space for situations players can be in, a minimum of 10^56 unique states, compared to 10^47 for Chess and 10^14 for a common Poker variant
Their strongest AI, DeepRole, evolves strategies through counterfactual regret minimization. It was tested against bots that play randomly, a LogicBot programmed to avoid making teams as Resistance that must have Spies, and bots that derived a strategy from a Monte Carlo Tree Search. The DeepRole AI proved superior to the other AIs.
DeepRole has a 65.6% win rate for Spies for 5 DeepRole players playing against themselves, compared to a 59.7% win rate for Spies in 5 player human games, and outperforms a replacement human player in games with either 4 bots or 4 humans. (it’s hard to assess the quality of human players in their games, and I think strategic superiority is difficult to test, discussed later)
The sample games they provide also show strange behavior from the DeepRole AI, sometimes making moves I would consider suboptimal.
Taking actions the whole table knows can never benefit Resistance, as both Resistance and Spies.
Rejecting the fifth proposal. Rejecting the fifth proposal is a game-losing condition for Resistance. In one of their sample games, the Resistance team loses as a result of DeepRole Resistance bots voting this way.1
Proposing and approving teams the entire table knows are bad. This can happen when a player asks to repeat a failed team, or includes a failed team as a subset.
Rejecting teams the entire table knows are good. This can happen in situations where if any Spies had been on the team, the game should already be over due to the Spies being able to fail a third mission.
Proposing and voting for teams that can only be good if you are a Spy. In a 5 player game, this is proposing or voting for any three person team without you.
Taking actions as a Spy that are never optimal for Spies. In one game, the AI succeeds as the only Spy on a mission that ended up being the third successful mission, forcing the game to a Merlin guess.
It sometimes makes sense to bluff a success on early missions or when paired with another Spy, but there is zero benefit to bluffing over failing here, as the game is over the moment the bluff could have any impact.
Without generating an explicit proof, I suspect that there is a strong incentive for players to optimize away from taking actions that the entire table knows cannot possibly help a player on the Resistance team (1.1-1.4 above), as Resistance controls the majority and wants to punish players acting like Spies. A possible weak rationale is that the DeepRole AI could be attempting to bait the Spies into taking aggressive Spy actions by improving the chances they’ll succeed, or communicating with itself using wasted actions, but given the Spy play which is provably suboptimal, I think it’s more likely the DeepRole AI simply makes some errors at this point.
The paper attempts to justify an example of 1.2 above as Merlin trying to act like they’re not Merlin (their AI also does this as base Resistance which is less explainable), but I’d argue this is just a mistake two-levels deep: a Spy AI trying to guess who Merlin is should ignore a player proposing teams it knows the entire table will reject, this is essentially engaging in another form of “cheap talk” that doesn’t influence the game, a move which is equivalent to saying “I am a Spy.” Merlin acting like base Resistance to bluff the Spies makes sense, I don’t think Merlin (or base Resistance) acting like a Spy should fool anyone.
I haven’t tweaked their code to test whether avoiding these behaviors would improve outcomes, but their results show a Spy win rate higher than games with human players, and my intuition is that perfect play would not involve behaviors like 1.1-1.4 where Resistance players appear to sabotage their own team, as it neither helps to win the game or provides a credible bluff.
The ProAvalon website describes some of these AI behaviors as being bannable offenses if done by a human player, as they are considered to be game-throwing. At the very least, I would predict that an AI which did not make visibly poor Resistance plays would fare better alongside human players than one that did, holding all else equal, and that allowing a third mission to succeed as a lone Spy should fall out of a dominant strategy long-term.
Update: Jack Serrino, one of the authors of the 2019 paper graciously weighed in with some thoughts, which I’ve shared in the footnotes2. The short version is that given how it optimizes, DeepRole will sometimes behave strangely by human standards in cases where it’s confident it’s won or it’s confident it’s lost, stalling when it knows the right team, or effectively giving up. I think this helps explain some, but not all of the unusual behavior. (rejecting the fifth proposal on mission one seems like it should still have been an undetermined game)
Cheap Talk, non-binding commitments, and Cryptographic Merlin
Both the DeepRole AI and my own implementation ignore “Cheap Talk” as part of simulating the game, but my intuition is that optimal play for Resistance involves heavy use of cheap talk. Consider the following scenarios:
Ambiguous social cues. Those of us who aren’t robots might say this is the whole point of the game! Free-flowing conversation can give Merlin the opportunity to drop hints that Spies might miss, give players a sense for what teams are likely to be approved, and allow players to catch each other’s tells.
Non-binding commitments. Players can make non-binding commitments that they are incentivized to keep to maintain trust at the table, improving the public information about possible outcomes of votes and making it easier for Resistance to form consensus. A player can announce they won’t vote for any team with another player they distrust on it, making it clearer which proposals are unlikely to be approved. Another common case is that after the table has heard the fourth proposal, the player who would make the fifth proposal can make their team known in advance to make the trade-off between the two proposals clear. All of this reduces the role of luck in the Resistance team reaching consensus.
In principle, you could introduce meta-based non-binding commitments in a simulation where AIs are communicating with each other, if you start with a context where players are judged as Spies and punished for deviating too far from their stated commitments, and include mechanisms to update their commitments to allow players to converge on a consensus team.Simulating private communication/Cryptographic Merlin. In a degenerate case, unrestricted use of “cheap talk” could create an equilibrium strategy where the Resistance team can use Merlin’s information to guarantee a successful team without revealing Merlin’s identity, in a game where Merlin knows every Spy.
Given the existence of a shared public communication channel, a Resistance player could suggest that all players make use of a public/private key encryption algorithm, generate a public/private key pair for themselves, and announce their public keys over the shared public channel.
All players are then encouraged to send padded messages of identical size to each other player, encrypted using their own private keys and the other player’s public keys, which are decrypted in turn. Merlin uses this opportunity to share their information about the Spies with all Resistance players and sends useless messages to Spies, while Resistance players send useless messages to everyone. Spies learn nothing from Resistance and Merlin, Resistance learns the identity of the spies from Merlin.
The Spies could attempt to counter this by also claiming to be Merlin and proposing a set of Spies to the other players. In a 9 player game with 3 spies, this could result in scenarios where Resistance can easily tell who Merlin is (out of the 4 people privately claiming to be Merlin, only 1 accuses the other three), or scenarios where Merlin is ambiguous. (out of the 4 people claiming to be Merlin, 2 or more accuse the other three).
In the worst case, there is public knowledge that doesn’t depend on Merlin’s identity that 3 specific players are good which the Resistance team can build on, improving their odds even if they ignore information that traces back to Merlin’s identity. And with a bit of randomness, Resistance can use their budget of two failures to test theories they may already have ruled out about who the Spies might be, forcing Spies to a Merlin guess after three successes.
Using full-blown cryptography to break the game is obviously a degenerate example, but I think there is a conceivable Nash Equilibrium where the Resistance team is aware of this strategy, exploits it ruthlessly, and no one has an incentive to switch off it as it always benefits the majority.
I’ve read about some groups allowing private conversations between players, which could lead to breaking the game as described above if the Resistance players are willing to waste everyone’s time having filler conversations to get all necessary information out without it being clear who communicated what. I maintain a house rule of not allowing private or non-universally-intelligible communication when I play, but a strategy like the above could conceivably evolve with the use of an unrestricted communication channel between AIs which could be difficult to enforce against: the ceiling on what you can coordinate with “Cheap Talk” is very high.
Update: Jack Serrino let me know that Paul F Christiano has done a similar analysis, which reaches similar conclusions about the power of private communication.
AI Tournament Overview, and basic stats
All bots coded for my AI tournament were considerably less sophisticated than DeepRole; no participant coded an implementation that evolved a strategy through machine learning or repeated training, but through a combination of human-intelligible rules and randomness which we tested against different implementations in Monte Carlo simulations. I’m confident that none of these implementations are close to optimal, and likely not even close to DeepRole’s skill level, but they can provide some insight into what human-intelligible strategies are most effective.
I started participants with a RandomPlayer implementation similar to the 2019 paper’s LogicBot (everything we did was developed without reading the 2019 paper). RandomPlayer avoids taking a few actions generally don’t make sense for a base Resistance player:
It never proposes or supports any team which is guaranteed to fail given public knowledge
It tries to repeat or build on a recently successful team
This is optimal against naïve Spies that always fail, but questionable in other contexts. My intuition is that the benefits of changing successful teams (outside of the two fail mission) are usually outweighed by the risks of switching off a good team. There may be outlier scenarios, like Percival scrapping a team by executive fiat that has to contain both Merlin and Morgana.
It always votes for the fifth proposal to avoid losing the game
It picks randomly between feasible options when not constrained by those points.
As a Spy, it fails missions when it’s alone, and rolls an N-sided die (is a coin a 2-sided die?) when deciding whether or not to fail on a mission with N spies on it
This is probably not optimal as a baseline, since waiting to fail on larger mission sizes gives the Resistance team less information.
These are the win rates for the Resistance team with RandomPlayer playing against itself in base Resistance (no Merlin/Assassin), and Avalon (where the bot doesn’t take advantage of Merlin’s information at all):
Some basic insights from this:
Despite avoiding some of the unusual failure modes of DeepRole, the RandomPlayer bot clearly underperforms DeepRole in its ability to coordinate a Resistance team or exploit information in the 5 player Avalon variant.
If all you do is avoid missions that are provably bad and repeat successful missions, you can win by luck a non-trivial share of the time, suggesting a skill floor of 14% to 30% Resistance wins depending on your game size and variant. There’s also presumably a skill ceiling at 66.6% for the Resistance team at 5 players, as the Spies can guess Merlin randomly and be guaranteed to win a third of the time.
This also demonstrates that the game is most favorable to Resistance at 5/7/9 players, where mission sizes and the ratio of Spies to Resistance are most favorable. Our group tends to add additional mechanics like Lady of the Lake or the Trapper module at 8 or 10 players, to nudge the balance back towards the Resistance team.
Also for reference, for a while I recorded data on games I played in real life with different modules and game sizes, in a group with a fairly stable group of players. None of these variants were covered by either simulation discussed in this article.

Plot Cards is a variant for base Resistance where team leaders get cards every mission to gain information, like learning a player’s team.
“All Roles” includes Merlin/Assassin/Oberon/Mordred/Morgana, with Morgana serving double duty as Assassin when necessary
The Trapper module puts an extra person on each team than would normally go, and allows the team leader to privately view and exclude a player’s success/fail mission card after it’s played. This makes the game easier for the Resistance team.
The Lady of the Lake module allows the current player holding a token to learn a player’s team by passing a token around, after missions 2, 3, and 4. This also makes the game easier for the Resistance team.
Lastly, these are Resistance win rates from ProAvalon.com from online Avalon play, which comes with some caveats that the dataset doesn’t distinguish variants used to balance the game, the online game has properties like a public vote history that don’t carry over to real life play, and these stats may not exclude bot play:
10 players having the highest winrate is counter-intuitive, and may be due to self-selecting variants for balance; a 10 player game is strictly harder for Resistance than the 9 player variant due to the presence of an additional Spy.
Comparison of AI Strategies: Bot Types
Back to the tournament, the AIs we created compete in interesting ways, with some optimizations and outright bugs that make comparisons tricky. To pull out some insights, I’m going to comment on how strategies from different bots perform when layered onto the RandomPlayer.
SelfPromotingPlayer
Always includes itself on team proposals (outside of cases where it’s provably a Spy and it knows the entire table will reject), and is more likely to support missions when it is on the team.
AggressiveMerlin
Acts like the SelfPromotingPlayer normally, but as Merlin, always votes and propose teams “correctly”, never supporting spies
MerlinDetector
Acts like the SelfPromotingPlayer normally, but as the Assassin, chooses who to assassinate as Merlin by picking the player who made the fewest mistakes in voting and picking teams
SneakyMerlin
Combines the strategies of AggressiveMerlin and MerlinDetector normally. If it is currently the most accurate player at opposing the Spies, it makes an incorrect decoy vote or proposal so someone else ends up as the most likely Merlin target at the end of the game.
ArmanBot (you can also call it a TrustBot)
I coded all of the above implementations for this analysis with insights from other strategies, but this bot is taken unmodified from our AI tournament champion and fellow Avalon enthusiast Arman Erfanar, who also reviewed this post.
His bot tracks a trust score3 for each player, which penalizes players for supporting bad missions, being on bad missions or not being on successful ones, and disagreeing with the bot’s own votes. It tries to pick the players with the highest trust scores for votes and teams.
As a Spy, it tries to stick to the above meta by favoring players with good trust scores, while also trying to push missions with itself, and enough Spies to fail a mission. (You could call this an AggressiveSpy strategy, where Spies use their info to push hard for Spy teams, and most of the MerlinDetector points from the discussion below would apply here)
Amazingly, this bot makes very little use of Merlin’s information and makes NO attempt to guess Merlin beyond pure randomness, and still beats every other bot implementation.
Comparison of AI Strategies: Basic Merlin Strategies
The below table is calculated for 10 player Avalon games with only Merlin and the Assassin as special roles, averaging outcomes across 500K games, and showing overall win rates. (Don’t worry if you can’t read the table, the discussion below is clearer than the table itself)

This table has different properties than you may have seen in my other posts: winRates on either side of the diagonal are not the inverse of each other as the bots can end up on the same team as the other bots. A bot playing against itself also doesn’t win 50% of the time because the teams are not of equal size and may not have equal winRates. I would summarize as saying the bots improve in quality as you go right or down. RandomPlayer is superseded by SelfPromoting, AggressiveMerlin, MerlinDetector, SneakyMerlin, and then by ArmanBot.
The interaction between some of these bots, like MerlinDetector and AggressiveMerlin, is understated by these overall win rates which average out impacts on both teams because team composition is mixed between the bots. When a single bot type controls an entire team, the differences can be quite large. The table below shows Resistance win rates for the bot in the row when playing the bot in the column as Spies, in fully partitioned games by team.

To summarize:
SelfPromotingPlayer will only win as Resistance 15.1% of the time against comparable players who are Spies. Without making use of Merlin’s information, these bots do poorly as Resistance.
AggressiveMerlin players bump that Resistance win rate up to 43.1% of the time against SelfPromotingPlayers who are Spies. A single player always voting and proposing teams correctly as Merlin causes a big swing in win rates over the course of the game, even when no other Resistance player is paying attention to who might be Merlin, and just leaning into successful teams.
When AggressiveMerlin players are pitted against MerlinDetectorPlayers as Spies, AggressiveMerlin players only win as Resistance 4.2% of the time due to the Spies correctly guessing Merlin. AggressiveMerlin bots playing Merlin are essentially annihilated by an Assassin bot that simply tracks the most accurate Merlin candidate.
If you upgrade AggressiveMerlin to SneakyMerlin, where it only votes and proposes correctly when it’s not the most suspicious player to be Merlin and pit it against a MerlinDetector Spy team, its Resistance win rate settles in at 21.1%.
Essentially, at this level, Merlin can only act when it’s operating within the level of random noise from the other players. If someone else is voting really well, Merlin has lots of cover to act, but if everyone else is voting badly, Merlin has to vote worse and wait for an opportunity to make a difference. 21.1% is much worse than a 43% winRate when Merlin can act openly without fear, but still better than a baseline 15.1% win rate when no one makes use of any secret information.The ArmanBot annihilates every other bot type when playing as Spies solely due to being an AggressiveSpy player that pushes teams with Spies; there would be a similar dynamic of a detection arms race with bots trying to detect this behavior (which the ArmanBot itself does). Its Resistance win rates are more complicated, discussed later.
The problem the SneakyMerlin bot is trying to solve is comparable to the challenge of how to make optimal use of the intelligence from cracking the code for the Enigma machine in World War 2, as dramatized in the movie The Imitation Game, and the Neal Stephenson book Cryptonomicon. If Merlin exploits their inside information aggressively, they will tip off their enemy to what they’re doing, so Merlin has to act in ways that have plausible deniability and don’t make the source of their information clear.
An important caveat: The bots above are mostly proof of concept implementations, I think the algorithms are very exploitable (if you know Merlin is trying to hide, do you just always take the second most likely player, or detect votes made to duck detection?), but I would suspect that optimal play approaches something similar in a mixed strategy, where Merlin is in an arms race against the Assassin to hide their actions, and the Assassin is trying to guess who is acting within the threshold of random noise.
ArmanBot: In a league of its own
The ArmanBot’s superior win rates compared to the competition understate how well it does in a partitioned matchup where it controls all of one team: this bot wins around 62% of the time as Resistance against a team of SneakyMerlin or MerlinDetector players running the Spies, while making zero use of Merlin’s knowledge.
The ArmanBot has two big advantages going for it:
Its trust score algorithm does a reasonable job at weighting evidence for which players are most likely to be Spies or Resistance, given their history on teams, proposals, and votes, where it appears to be superior to random play and catches aggressive spies.
Playing against itself, it achieves an impressive 31.1% winRate as Resistance. However, the bulk of this edge is due to its Spy-detecting behavior matched against its own AggressiveSpy play. The ArmanBots Spies are aggressive about promoting Spies in ways the Resistance ArmanBot catches, and further refinement might change this win rate. (similar to how AggressiveMerlin is caught by MerlinDetector). If you change the Arman bot so the Spies don’t exploit their knowledge to push missions with Spies, the Resistance winRate only hits 18.1%. This is better than any other bot does without using Merlin’s info, but it suggests most of the ArmanBot’s edge at Resistance play against itself is due to detecting Spies who exploit their information.It overwhelmingly favors copies of itself in proposing and voting on teams, by trying to find other players that vote like it does.
The presence of multiple ArmanBots in a game make the team with the most ArmanBots more likely to win, and in a purely partitioned game, Resistance ArmanBots have an excellent chance of locking onto all the players acting like them and settling on a united team.
The first point is just being smart, the second point sort of breaks how I was scoring the tournament, through no fault of Arman himself or anyone else. This was an unintended consequence neither of us saw coming. A dominant strategy for how I was running the tournament is to find bots that act like you do, and promote their success, as you could be finding your teammates in a partitioned game, and promoting copies of yourself is likely to boost your overall win rates otherwise.
Another way to look at it is that the Arman bot has two levels of social norms:
Punish players who end up on failed missions or support failed missions.
Punish players who deviate from its preferred norms for picking teams, which effectively means punishing players who don’t exactly follow its logic for point #1.
This pattern reminds me of a discussion of how social norms operate in a recent book on game theory called Hidden Games:
In many contexts, a group with a social norm will punish other people who deviate from that norm
After that behavior is observed, the group will punish people who didn’t punish other people for deviating from their norms
After that behavior is observed, the group will punish people who didn’t punish people for not punishing people for deviating from their norms, and so on.
In the specific context of this game, the ArmanBot has some provably smart insights about lowering the odds of Spies ending up on your teams, but imposes a set of strict team norms that make it semi-hostile to players who think differently than it does. This is part of the reason I think it’s difficult to test how truly optimal a bot like DeepRole is. An unusually effective strategy seems to be: “the definition of someone trustworthy is someone who thinks and acts like me.”
The profound philosophical and ethical implications of this insight, and how to re-organize all of human society so that we are not destroyed by viral social norms spreading intolerance in the name of virtue, will be left as an exercise for the reader.
Conclusion
I’d summarize my thoughts as follows.
The DeepRole AI is able to solve some impressive coordination problems. While some reporting on the AI described it as superior to human players, I’m not sure if it would beat top human players yet, as the AI can be observed to make at least one type of clear mistake, and doesn’t settle on meta points beneficial to the team controlling the majority. It also doesn’t handle social aspects of the game that can give an edge to human players.
Related, I believe that “Cheap Talk” can improve coordination between human players, and is an important part of modelling a game like Avalon (my own implementation also excluded this for simplicity).
Both teams benefit from making use of hidden information without being obvious about it. An aggressive Merlin when the Spies are not paying attention to the votes and proposals can have a surprisingly large effect on Resistance win rates in the context of generally naïve play, boosting win rates from 15% to 41%, but this falls off if Merlin is forced to act within the level of random noise, only raising win rates to 21%.
Similarly, the ArmanBot’s aggressive Spy play leads to large margins, which become negative against the ArmanBot’s own Spy-detection logic. Spies face a similar dilemma to Merlin, where they can improve their chances by aggressively favoring teams which match their interests, but a smart bot can detect this behavior and shut them down.
Exploiting a source of information without revealing that you’re exploiting it is an interesting problem explored elsewhere.Weighting your trust in players based on their past actions using some probability appears to raise the general quality of Resistance team play.
There are likely metas which are local maximas, as punishing players for deviating from your preferred team norms or just acting differently than you would, is a surprisingly effective strategy.
Areas I’d be interested to see explored by future research, from myself or others:
What optimal Resistance gameplay looks like without Merlin in the game. The ArmanBot proves you can do better than just avoiding teams guaranteed to fail and repeating successful teams, so there’s probably some probabilistic solution here.
What optimal exploitation of hidden information without being caught looks like. Merlin play which exploits information while hiding behind the level of noise from other players appears to be effective, Spy play could be similarly refined, but there’s probably a high skill ceiling here, which could also be influenced by other Resistance players trying to guess and support Merlin themselves.
How to avoid the problem of bots unfairly favoring copies of themselves. If you have two types of bots who both enforce different arbitrary voting norms, both bots might underperform a replacement bot of the other type in a game already filled with bots of the other type.
The 2019 paper briefly discusses testing that their bots were resilient to evolutionary invasion. I haven’t dug in deeper yet, but it’s possible this problem is already explored elsewhere.How to refine an agent like DeepRole to avoid obvious mistakes, and understand its gameplay.
How different Resistance/Avalon variants and team sizes compare in team balance, and what strategies emerge that are unique to a variant.
Incorporating the “social” dynamics of the game into simulations and AI gameplay through language models or other tools. This might allow AIs to engage in true games with humans, or evolve more advanced strategies using communication.
The game is strategically deeper and more entertaining in person with all of the social dynamics. I personally favor layering on a number of extra roles (Percival/Oberon/Morgana/Mordred) to add to the complexity of the information being tracked and the problem-solving everyone is able to do.
If you’re interested to get started, the fantasy themed Avalon is a better base set than the sci-fi themed Resistance, which doesn’t have the role-based gameplay which makes the game most interesting. The Avalon roles have been ported to the Hidden Agenda expansion to Resistance which also includes the Trapper module, enabling larger team sizes and the exclusions mechanic. I have not gotten much use out of the Hostile Intent expansion, other than the Inquisitor/Lady of the Lake module I had the cards for anyway.
I personally own all variants of this game, but I think Avalon is the best value for your money to start, and I think the game shines at 7 or more players. I don’t yet have a perspective on the related game, Quest, but would be interested in other people’s experiences.
Opinions differ on this. In real-life games, some strategies endorse the concept of “protest votes”, where you vote against the fifth mission to signal how much you really dislike it.
In the context of that meta, I might not have a choice but to play along myself: the game you’re playing is largely determined by the other players.
However, I personally think a Resistance player can communicate the same intent without taking an action that risks losing the game, as the action they’re taking doesn’t actually map to their intent. I think you could sing a few bars of “I’m Against It!” from the classic Marx Brothers film Horse Feathers for the same effect.
And generally, I think the majority of players who find themselves on the Resistance team in a given game should be able to speak up and say “Hey, maybe let’s avoid taking actions that can only help Spies, and vote against people who take those actions”, as a baseline for gameplay.
Additional commentary on DeepRole from Jack Serrino, one of the authors of the original paper:
”I found your write up very insightful. So in depth! So many bots! It would have been cool to have all of the bots play w/ DeepRole online - it's unfortunate ProAvalon had to take it offline, but ultimately understandable.
Re: off-meta/bannable moves - especially in cases where DeepRole considers the game totally lost (or totally won), it will often make plays that seem nonsensical - from its perspective, it doesn't matter what it does. Since it assumes other players will play the same way it does (modulo differences in information), when the result of the game is "obvious", it's confident it can pull the win at some point.
This isn't really how people play! People would prefer to win earlier than later, and people certainly don't want others being bad sports about things, gracefully losing instead of dragging things out. This would imply we want to bias DeepRole to quicker outcomes rather than slower ones.
Many (most?) RL agents have an explicit discount factor which will bias them towards winning quickly. DeepRole does not have this explicitly, perhaps a partial explanation of the weird behavior.
Or, perhaps it wasn't trained for long enough ;)”
More details on the ArmanBot’s trust score calculation if you want the specifics:
1. As Resistance, it only votes for teams if all players in the top half of trusted players, or are precisely the most trusted players if the team is a majority of all players.
2. As a Spy, it only supports teams that have the minimum number of Spies to fail the mission, no more no less, unless two missions have already failed, in which case it takes any team guaranteed to fail.
3. When proposing a team of size N as Resistance, it takes the N most trusted players, as a Spy it does the same thing, only it includes itself. (this might be optimal for Resistance as well, although without it, the bot has a property of converging on the same team from different perspectives, which influences outcomes)
4. Initialize SpyChance scores for Resistance players using the number of Spies divided by the number of players minus one. For example, in a 9 player game with 3 Spies, if you are Resistance, each other player has a 3/8ths chance of being a Spy
As Merlin, it appears to initialize spyChances at 1 or 0 based upon them actually being Spies, but these odds interact strangely with the algorithm, where Merlin consistently votes worse than base Resistance, considering that the MerlinDetector bot does worse than random chance against ArmanBot’s version of Merlin. In general, the bot’s Merlin gameplay does not appear to be a dominant factor in how teams are formed.
5. If a mission succeeds, every player not on the mission has their spyChance score increased by their existing spyChance score multiplied by the number of spies in the game divided by the number of people not on the mission.
For example, in a 9 player game, if the first 3 person mission succeeds, the 6 players not on the mission have their score increased by the initial value of 3/8ths multiplied by (3/6). I don’t think this maps to a true probability at this point, but somewhat tracks with the conditional information you’re getting.
Anyone who voted for the successful mission has their spyChance reduced by .01 multiplied by the mission number, and anyone who voted against has their spyChance increased by the same amount.
6. On a failed mission, every player on the mission has their spyChance increased by the number of fails divided by the team size multiplied by their initial spyChance.
Players have their spyChances increased or decreased with the same logic as mission successes, based on whether or not they were correct.
7. After a vote, it increases/decreases everyone’s spyChance by .01 based on whether that player voted with them or not.
This trust score calculation was worked out over a short period of time during a hackathon. It’s far from fully optimized, but even something this simple creates a number of interesting consequences for gameplay. The most re-usable part of it is probably catching AggressiveSpy behavior.
As extensions, I would be interested in making a bot with this approach less deterministic, refining its thresholds, and testing behavior without penalizing other players for voting differently than it does.
As shown in your tournament results, the ArmanBots get the highest win rate simply by analyzing other's behavior. They don't need Merlin or Perci to give any info by speech. So why bother cheap talk? If Merlin and Perci (and other res) are behaving well enough, naturally they will earn others' trust. As for the spies, in order to fail the missions, they unavoidably have to do bad things and will eventually be punished by the res. I believe relying mainly on team proposals, votes, and mission results, not being misled by the good actors and orators can help both AI and human players make better and more accurate decisions for their own team.
Super interesting analysis, thank you! I love Avalon but it's hard to get a group to play...most of my friends are not fans of the 'hidden identity' genre, whereas I love it. Probably why I love playing poker, too.